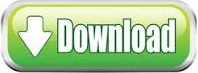
This approach, called the Strassen algorithm, requires some extra addition, but this is acceptable because additions in a computer take far less time than multiplications. Our goal is to find Z parameters so that the distance of two points in one coordinate system becomes as minimum as possible.But the mathematician Volker Strassen proved in 1969 that multiplying a matrix of two rows of two numbers with another of the same size doesn’t necessarily involve eight multiplications and that, with a clever trick, it can be reduced to seven. In the above formula, X parameters are P1 and P2 coordinates in the normal plane, calculated by multiplying P1 and P2 into the inverse of the intrinsic matrix (K).īased on the above formula, the X1 point will shift to the coordinate system of the X2 point. To compute the z parameter below formula is used:
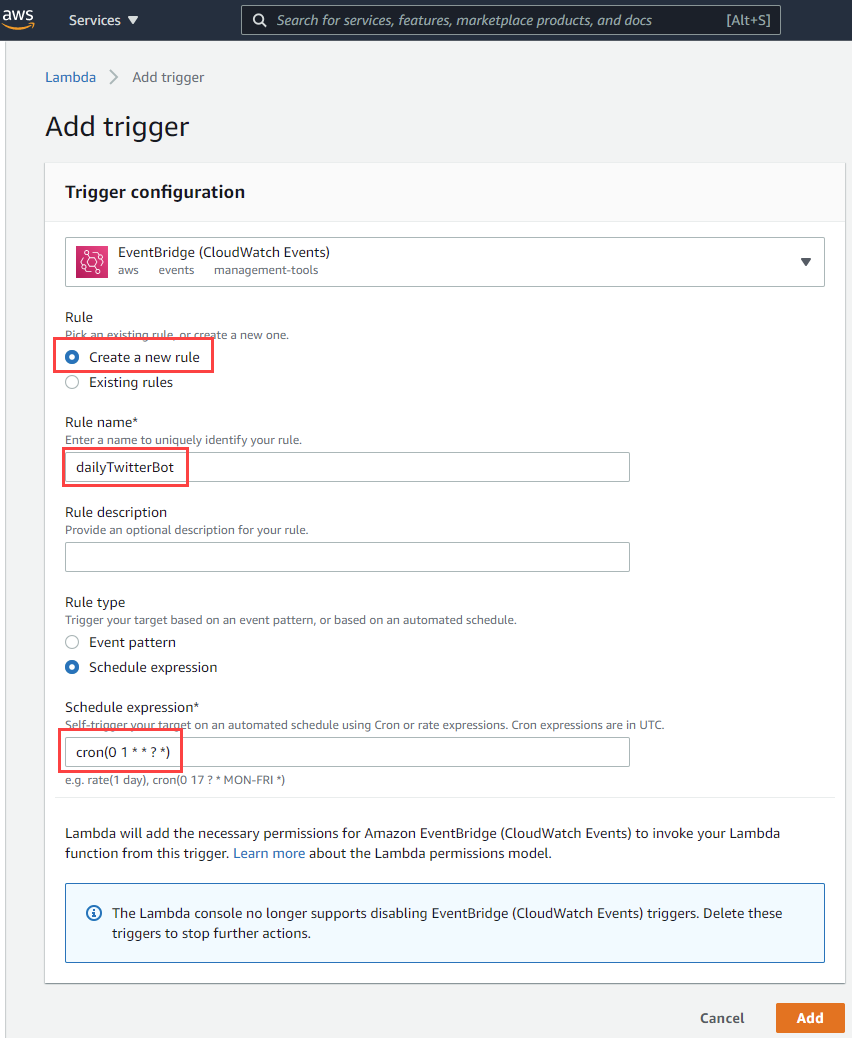
So to change the P1 camera coordinate, we need an extrinsic matrix, the camera pose, or the projection matrix found in the previous part. Since these two points indicate the same real-world point and are identical, changing the P1 coordinate system to the P2 coordinate system P1 should fall precisely on P2 (Based on pinhole camera models and equations). Two blue rectangles are the images that P1 and P2 were extracted from receptively. In this image, P1 and P2 are feature points pointing to an identical 3D point P located in the real world. To calculate the z consider the image below, When these points are in the normalized plane, we can use their x and y coordinates as the 3D x and y, so the only remaining item will be z because, as mentioned earlier, in monocular cameras, depth is missing. At first, we bring them to the normalized plane (If you are unfamiliar with the normalized plane, it is better to read about the pinhole camera model). Extracted feature points are all in the image coordinate system. Now that we have the projection matrix, we can estimate the 3D position of feature points using the “ Triangulation” method.
#MATRIX RSS BOT HOW TO#
How to estimate the feature points real position? Now we can use Singular Value Decomposition(SVD) to find the camera pose equal to the projection matrix or camera pose. If we need the fundamental matrix, we use this formula 𝐹 = 𝐾⁻ᵀ 𝐸 𝐾 ⁻¹ where E is an essential matrix. We can form the Essential matrix by extracting 𝑡 ∧ 𝑅. This matrix can be found using camera calibration methods. K is an intrinsic matrix composed of focal length and principal point. In the above equation, p1 and p2 are two matched feature points showing an identical 3D position of a point in real-world coordinates. They can be extracted from an equation named epipolar constraint equal to zero. Long story short, essential or fundamental matrices are needed to get the first pose of the camera. The calculations in this step are done based on epipolar geometry by extracting essential or fundamental matrices. We call it “Initialization.” Initialization is a crucial step in monocular slam because depth, scale, and the very first pose of the camera will be found. In monocular slam, since the depth of the images is not accessible, and the only thing we have is 2D images, the recovery of the three mentioned entities is hard and almost impossible. To show a camera or any point in the map (if the 3D map is used), three entities: X, Y, and Z, are needed.
__scaled_600.jpg)
Finding corresponding matched feature points that help us to estimate the camera pose (Rotation + Translation) is the second usage of them. Image matching helps us at first to know that the images are related enough to use them in the slam algorithm. How to find camera trajectory using feature points?Īfter extracting features, image matching is the most basic yet important thing to do. The block is part of the image with no changes or less change in light brightness. The intersection of two edges is called a corner. What are edge, corner, and Block?Įdge is part of the image where extreme changes in light brightness are seen in one direction. They have a better application than pixels. Research shows that edges and corners in the images can be considered feature points. Also, SLAM systems need stable features under different image visual changes like rotation, scaling, and brightness. The major problem with this approach is that by increasing the size of the image time complexity of the process will be increased, which is not good. The simplest one is written on Wikipedia, “a set of information related to computing tasks.” Another superb definition more specific to our discussion is “digital expression of image information.” Based on this term, every image pixel can be considered a feature, but there are some problems with this. There are different definitions to answer the question. These features make the map, and they are the main components. As mentioned earlier, the front-end in visual slam extracts feature points.
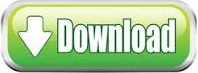